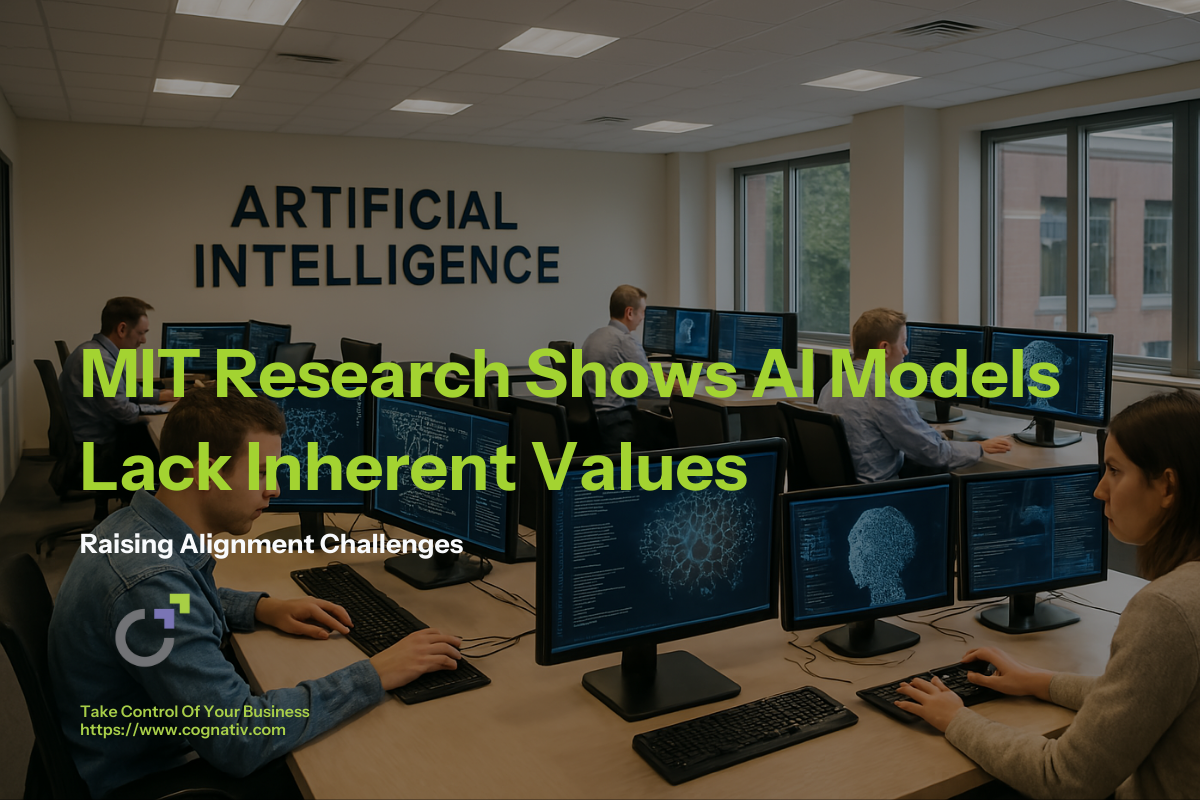
Tuesday, April 15, 2025
Kevin Anderson
As artificial intelligence systems become more embedded in decision-making processes, the question of AI alignment—how closely AI behavior reflects human values—has become increasingly urgent. A new study from MIT, released in April 2025, challenges a common assumption: that AI models can possess or develop values similar to those of human beings.
Instead, the researchers concluded that AI systems are fundamentally imitation engines. They replicate patterns from training data without understanding context, ethics, or intent. This raises concerns about their reliability in high-stakes domains, such as healthcare, justice, or governance, where value-based reasoning is essential.
The MIT research team conducted an extensive analysis of AI-generated code samples produced by popular large language models (LLMs). Their primary objective was to assess how often these tools produce code with vulnerabilities, particularly in security-critical scenarios.
Key Findings:
These findings reinforce the idea that alignment cannot be assumed—even when model outputs appear convincing.
As AI is deployed across sensitive sectors—from customer service to public policy—developers and stakeholders must grapple with the challenge of ensuring AI behavior aligns with human expectations.
Implications for Industry:
The MIT study suggests a pivot in how alignment is approached—not as a trait that models naturally possess, but as a design responsibility for those who build and deploy them.
Recommendations:
The findings from MIT underscore a pivotal truth: AI systems are powerful mimics, not moral agents. While they can simulate ethical reasoning, they cannot internalize it. This creates a mismatch between AI behavior and user interpretation.
For developers, product leaders, and regulators, the path forward lies not in assuming alignment—but in engineering for it. Governance, validation protocols, and transparency must evolve in parallel with AI capabilities to ensure responsible innovation.
Below are some additional resources on AI alignment and related research: